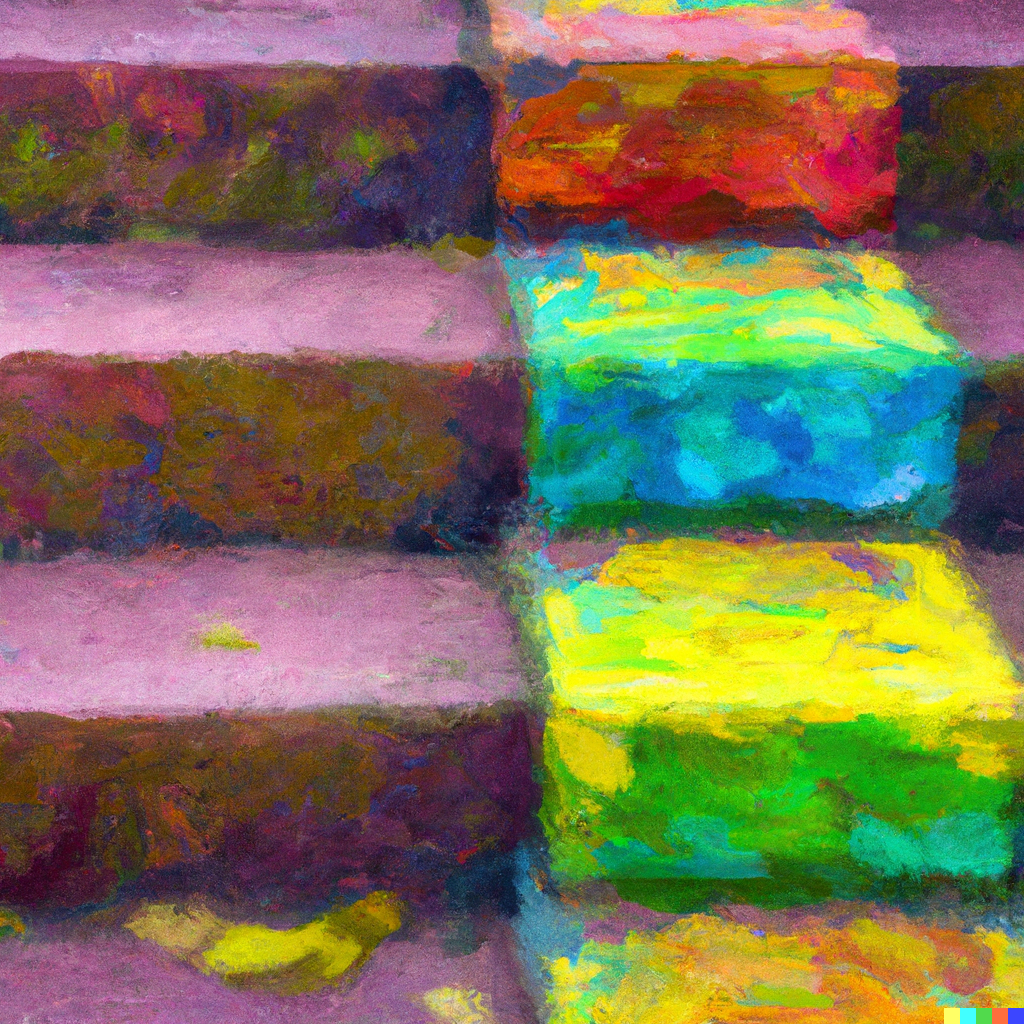
S32’s Wesley Tillu: Investing in the Age of AI Acceleration
AI adoption is accelerating within enterprises, creating a growing opportunity for application software companies....
AI has a long history. The Turing test was invented in 1950 and since then ML has experienced winters and summers. We are currently in a ML boom that began with the transition to Deep Learning starting in 2010. Since then we’ve experienced three ML infrastructure phases.
The first wave of ML infrastructure from 2010 to 2015 was reserved for a select few. In the beginning academics and researchers like Stanford’s Fei-Fei Li who created ImageNet and Ian Goodfellow who invented Generative Adversarial Networks (GANs) advanced training data sets and model’s capabilities. Businesses that had the finances and resources to take advantage of these advancements in Deep Learning were tech-forward, large companies like Google, Facebook, LinkedIn, and Netflix. They could hire PhDs and spend millions building internal ML infrastructure tooling. Leveraging ML to improve product experiences was a competitive advantage generating revenue lift so these corporations didn’t wait for third party vendors to emerge, but rather pushed the bounds of infrastructure themselves. These public companies became famous and well-regarded for their sophisticated ML teams and internal platforms.
The second wave of ML infrastructure, from roughly 2016-2020, led to the rise of ML infrastructure vendors that democratized access to tooling. During this phase product teams had real-world examples from the hyperscalers of how ML could improve user experiences. They started thinking creatively about how ML could be applied to their business. However, most product teams didn’t have the resources like time, talent, and expertise to build platforms themselves, which was often a multi-quarter, multi-million dollar effort, assuming they could even hire the team to make it happen. They needed third-party tooling.
Luckily, creators of some of the large scale ML systems were entrepreneurial and cloud service providers noticed the market opportunity. We saw a wave of vendors emerge to help fill the tooling gap. This included the rise of end-to-end ML platforms like AWS Sagemaker as well as specialized solutions like Tecton and Weights & Biases. Individuals implementing and leveraging these solutions sat in the data and data science domains. Professional specializations emerged, including ML engineer, MLOps, Data Scientist, and Data Engineer — each tasked with different responsibilities to help teams develop, test, and bring models into production. The development to production cycle was happening outside of the large technology companies, but teams were mostly training a model from scratch, a time-consuming and compute intensive process, which often took quarters.
The current, third wave of ML infrastructure further abstracts ML practitioners from core infrastructure. The emerging tooling is no longer simply focused on filling a void in the market but rather optimizing the experience. New solutions focus on ease of use, ergonomics, performance, and cost.
One reason new tooling focuses on user experience is because algorithms are more accessible and have advanced significantly. Model hubs like Hugging Face allow teams to more quickly compare and choose algorithms. Simultaneously, we’ve seen the emergence of foundational models that are available via open source and API. Fine-tuning these models with domain-specific data takes a fraction of the training time compared to training a model from scratch. Moreover, inference APIs make it possible for teams to easily integrate NLP, audio, and computer vision models into their products without training or fine-tuning. Examples include OpenAI’s GPT-3, Cohere.ai, AssemblyAI, Stability.ai, and Deepgram.
Today it is easier to go from 0 to 1 with ML than the past waves. Tools are simplifying and accelerating the process. Improved ergonomics means individuals do not need to be ML specialists to be ML practitioners. While cost remains a factor, ML infrastructure is starting to be abstracted enough that software engineers can become involved in the process. During our research, we often come across product engineers who are now fine-tuning models or leveraging inference APIs. This is a huge shift over the past two years. We have been excited about ML solutions since 2016 and like that the current wave of ML infrastructure further broadens ML’s accessibility. This is just the beginning.
Look for startups that help companies reduce costs across AI/ML implementations. “Performance and cost continue to be top of mind,” says Myers. “NVIDIA A100 GPUs are expensive so ML optimization startups have cropped up to eliminate inefficiencies at the algorithmic and systems levels across training and inference. Serverless ML runtimes like Modal allow users to train and deploy models for inference without having to configure or manage underlying infrastructure. These tactics help reduce infrastructure utilization and Total Cost of Ownership (TCO).”
AssemblyAI, Co:here, Deepgram, Hugging Face, OpenAI, Stability ai, Weights & Biases, Tecton
AI adoption is accelerating within enterprises, creating a growing opportunity for application software companies....
Carli Stein, Investor at M12 focused on AI startups across the stack, explores how smaller language models, robust security tools, and improved testing methodologies will enable broader AI adoption....
Vertical AI SaaS presents a prime opportunity for startups, fueled by increasing customer dissatisfaction with legacy tools. Karthik Ramakrishnan, Partner at IVP, assesses opportunities for startups to disrupt traditional workflows that offer tremendous market potential....